STEP Consulting and its partner D3S (Data Science Software & Services) support industrial actors to re-invent.
Digital Costing
Digital Costing applied to detailed parts
Context and Objectives
- automation of technical cost drivers extraction from Engineering data (3D & 2D)
- automation of cost target calculation with parametric formulas, defined by technology
Results
- basic parts from 3D models for the A350 family
- tubes & pipes from 2D drawings for the A320 family
- extraction of technical cost drivers : dimensions, weight, material, number of bendings, holes, weldings…
- integration of parametric formulas for several technologies : machining, bending, profiles, assembly, tubes & pipes, ramps …
Digital Costing applied to complex mechanical assemblies
Context and Objectives
An helicopter manufacturer wants to deploy the digital costing approach on its products, but the formulas, initially developed for structure parts, are unfit to cover specific parts, especially from dynamics (gear boxes, rotors/stators, shafts…)
It is decided to develop specific formulas to address particular geometrical or process features as “add-ons” on standard formulas
Results
The main cost-impacting features are identified (sharp tolerances, toothings & splines, thermo-chemical & anti-fretting treatments…), with associated identification rules (recognition of patterns in 2D drawings…)
Despite the lack of robust data (low volumes of parts concerned, difficulty to segregate the process phases…), a calibration is developed with experts (costing, manufacturing, procurement, engineering…)
The results relevancy is confirmed on the concerned parts
Digital Costing applied to mechanical and electronical equipment
Context and Objectives
Equipment costing relies on supplier’s raw data which are difficult to gather and extract industrially
- 20 large suppliers ; 30 major systems (flight controls, hydraulic power, fuel, engines control..)
- About 100 mechanical and electronical equipment in “build to spec” mode
- Hundreds of electronic cards references and thousands of components (diodes, capacitors …)
Business in constant evolution with product innovation (electrification, automation of crew operations, …), new data-based services and key actors’ strategy change (Make or Buy, acquisitions …)
Our customer need to spend less time on data extraction and preparation and more time to bring added value in price negotiation, as a partner of the purchasing organisation
Results
A stand-alone suite is built and delivered
- Data structuring: user-friendly interface allowing assisted data exploration (automated recognition of data structures, invites for user’s action/input when necessary, learning/capitalization of new recognized formats)
- Technical cost drivers extraction: digital solution developed in python to feed cost models
- generation of bill of materials and cost drivers based on electronic board pictures recognition (deep learning classifier, regex algorithm ..) and semi-automated extraction from engineering pdf documents (vision algorithms)
- web scrapping solution to collect prices of standard electronic components from internet
- Cost model digitalization: ~40 cost models for majority of aircraft equipment
- functions (I/O, CPU, Power …) based on electronic components (diodes, capacitors …)
- printed circuit boards, electronic boards assembly and tests, computers assembly and tests
- mechanical organs (actuators, valves,…) ; energy converters (motors, pumps..) ; displays, sensors
From days with thousands of tasks, to a few hours to perform an equipment costing
- Eg1 : Costing of a full landing gear (full system composed of several equipment) with analysis of scenarios (footprint, production rates), done in 6 weeks instead of 6 month
- Eg2 : Costing of 4 MCU computers based on functional inputs (performance, …) allowing to challenge make or buy policy
Digital costing applied to Design to Cost (DtC)
Context and Objectives
A high percentage of aircraft recurrent costs is conditioned by architectural choices made early in the design process. Therefore, it is fundamental to be able, very early and with a good level of precision, to predict the final cost of detailed parts and assembly.
The stakes for an industrial company are double:
- Simulate financial impacts of several architecture options, in order to support design choices
- Manage the evolution of recurrent costs throughout the design cycle in order to anticipate any deviation from the target cost defined in the business case
Results
Definition and development of a methodology for the prediction of final costs (“predictive costing”), based on:
- The latest available version of the design as a digital model, regardless of the level of design maturity
- A data reference database allowing, by proceeding in similarity with the data of the available design, to estimate as accurately as possible the detailed design parameters necessary for the estimation of the final costs (e.g. technical characteristics of the elementary parts)
Development and industrialization of a digital solution allowing to frequently monitor the evolution of the recurrent cost, in absolute value and in comparison with alternative or older design.
Development of several views to help the design office identify the cost drivers and support the choices between alternative designs:
- Breakdown of costs by industrial stage, by technology and by nature
- Pareto of technical cost drivers
- Sensitivity analysis of the parameters
Simulation of “trade-offs” between several architectures, types of materials…
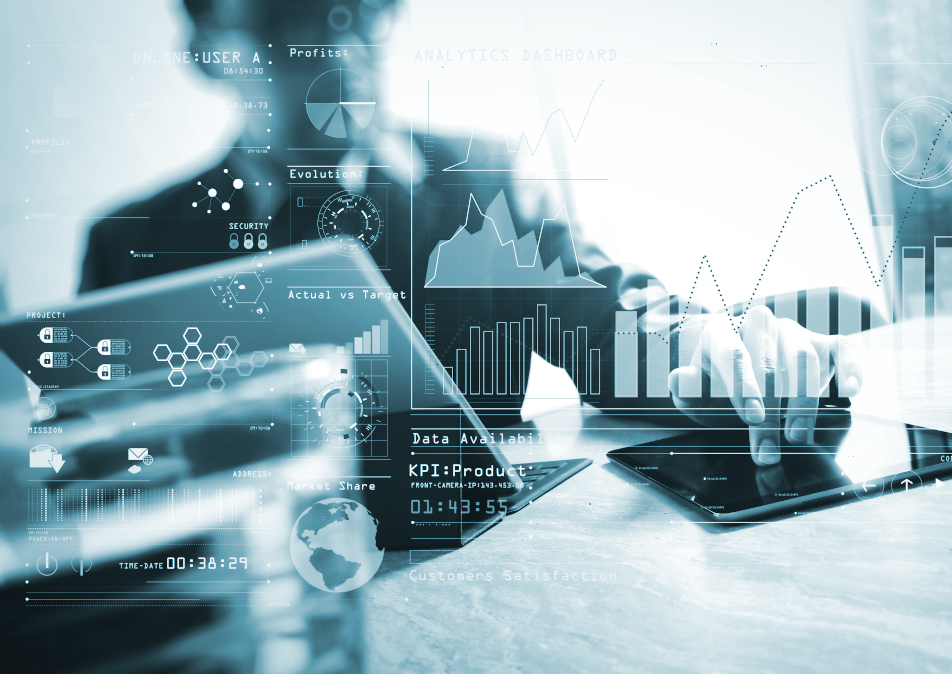
Finance function digitalisation
Finance Integration Tool (FIT) & unit cost prediction
Context and Objectives
An aero-structure provider (~2.2 billions euros revenues, ~7000 employees) wanted to identify the key drivers of its Buy in the Make spent as well as the levers of its improvements for a major program.
The project focused on two critical analysis:
- comparisons between actuals reports and BoM valuation at part number level;
- forecasts based on BoM valuation at weighted average price.
Results
Project is followed by the CFO at EXCOM level
Millions of work orders, supply orders, contract prices treated, tens of thousands of parts considered, thousands of BoM valued
Estimation of fluctuations due to work-sharing (+/-x%), overconsumption (+/-x%), good receipt price deviation (+/-x%)…
Interactive dashboard shared with 9 industrial controllers to visualize relevant data
Operating plan secured using BoM valuation and workshops with controlling teams
Predictive overdue payments and anticipation of cash recovery
Context and Objectives
A CAC40 industrial company, with more than 100 000 customers is facing overdue. In the past years, a cash collection process was implemented with more than 30 FTE calling customers to secure on-time payments.
This lead to -5% overdue but the head of credit management was willing to leverage available data to go one step further.
- accelerate cash recovery through predictive outcalls ranking
- improve efficiency, avoiding the use of excel files and manual drill-down into SAP
Results
The model is able to predict overdue payments at invoice registration date, with a good performance.
The solution is fully developed with MS SQL server (client constraint) and python
+20% productivity savings are demonstrated, when compared to the AS IS method (prioritized collection activities)
The industrial solution combines a MS SQL server, a python server and a direct push/pull connectivity with SaP BW (as a middleware)
Predictive pricing for a cable manufacturer
Context and Objectives
Large volumes of specific products are designed for one or two customers, with difficulties to set a proper price.
This situation can lead to lower margin on some deals, and a risk to lose large customers when prices are above market standards
Insufficient number of sales to use basic statistical peer pricing technics
Need more sophisticated machine learning algorithms to predict peer-consistent prices based on all product characteristics
Results
The project highlights major mispricing across customers, with possibilities to renegotiate futures contract
Design-to-margin opportunities are identified
A predictive tool is packaged and delivered, allowing sales to assess the price for any new product
Over a million euros of additional sales are estimated for a single plant, with opportunities to drop unprofitable products
Finance function digitalisation
Finance Integration Tool (FIT) & unit cost prediction
Context and Objectives
An aero-structure provider (~2.2 billions euros revenues, ~7000 employees) wanted to identify the key drivers of its Buy in the Make spent as well as the levers of its improvements for a major program.
The project focused on two critical analysis:
- comparisons between actuals reports and BoM valuation at part number level;
- forecasts based on BoM valuation at weighted average price.
Results
Project is followed by the CFO at EXCOM level
Millions of work orders, supply orders, contract prices treated, tens of thousands of parts considered, thousands of BoM valued
Estimation of fluctuations due to work-sharing (+/-x%), overconsumption (+/-x%), good receipt price deviation (+/-x%)…
Interactive dashboard shared with 9 industrial controllers to visualize relevant data
Operating plan secured using BoM valuation and workshops with controlling teams
Predictive overdue payments and anticipation of cash recovery
Context and Objectives
A CAC40 industrial company, with more than 100 000 customers is facing overdue. In the past years, a cash collection process was implemented with more than 30 FTE calling customers to secure on-time payments.
This lead to -5% overdue but the head of credit management was willing to leverage available data to go one step further.
- accelerate cash recovery through predictive outcalls ranking
- improve efficiency, avoiding the use of excel files and manual drill-down into SAP
Results
The model is able to predict overdue payments at invoice registration date, with a good performance.
The solution is fully developed with MS SQL server (client constraint) and python
+20% productivity savings are demonstrated, when compared to the AS IS method (prioritized collection activities)
The industrial solution combines a MS SQL server, a python server and a direct push/pull connectivity with SaP BW (as a middleware)
Predictive pricing for a cable manufacturer
Context and Objectives
Large volumes of specific products are designed for one or two customers, with difficulties to set a proper price.
This situation can lead to lower margin on some deals, and a risk to lose large customers when prices are above market standards
Insufficient number of sales to use basic statistical peer pricing technics
Need more sophisticated machine learning algorithms to predict peer-consistent prices based on all product characteristics
Results
The project highlights major mispricing across customers, with possibilities to renegotiate futures contract
Design-to-margin opportunities are identified
A predictive tool is packaged and delivered, allowing sales to assess the price for any new product
Over a million euros of additional sales are estimated for a single plant, with opportunities to drop unprofitable products
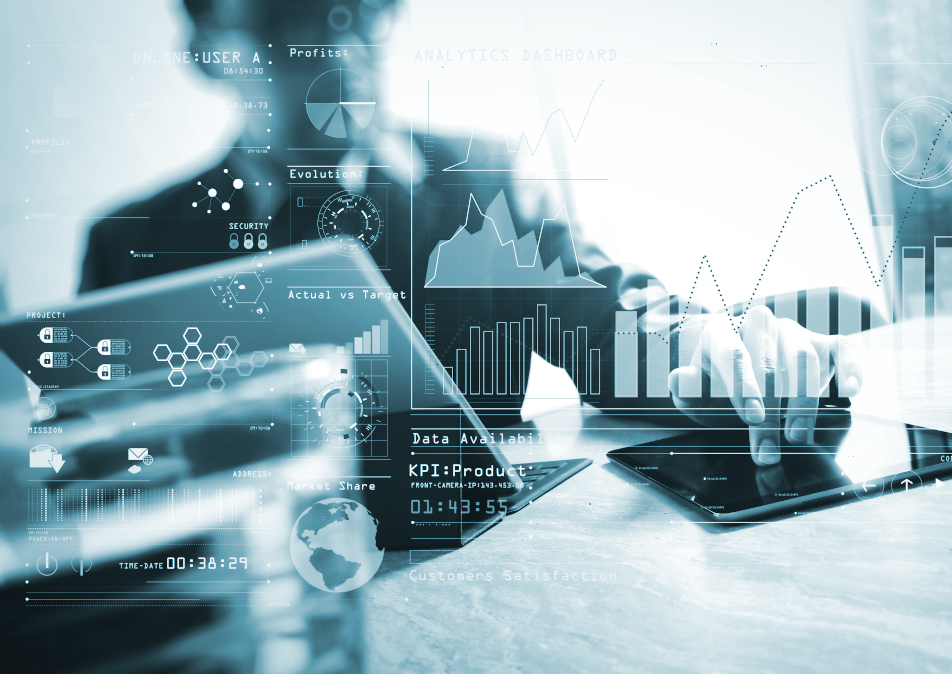
Manufacturing digitalisation
Manufacturing digital transformation
Context and Objectives
To face production ramp-up and preserve EBIT, the company needs to structurally improve operational efficiency ; decision is made to launch a digital transformation program, with purpose to:
- review the existing end-to-end process from design up to conformity attestation (through manufacturing engineering, logistics, manufacturing and quality)
- smooth data operations across all functions, eliminate data duplication, avoid manual operations, and reduce non-added value tasks
- accelerate the processing of parallel flow (non conformance, outstanding works)
- prepare future steps for the shop-floor
Results
Definition of 2014-2016 baseline for 3 plants
- about 3500 white collars impacted within manufacturing engineering, quality and logistics
- about 3000 blue collars impacted at shop floor level
About 40 major pain points highlighted along the end to end process, and a target of 25% cost reduction is agreed to be reached in 18 months
- 50 projects are defined and prioritized to answer the pain points
- local plateau organizations are created with ~100 people on projects and local governance
- decision board is in place every 2 weeks
Manufacturingoutstanding work prediction
Context and Objectives
An industrial ramp-up is threatened by the significant amount of work to be performed in final assembly line coming from upstream plants; 600 FTE additional workload.
Data to be analyzed are over 1 billion cells
Objective is to define action plan to reduce the level of outstanding work in final assembly line and to predict them in order to ease their management
Results
Diagnosis performed on major outstanding work contributors plant with identification of recurrent issues
Root causes analysis with text mining algorithms
Recovery action identified at operational level to tackle recurrent issues
Predictive model setup able to predict ~40% of the outstanding work at work order level
Optimization of raw material consumption based on nesting algorithms
Context and Objectives
Cost of raw material (aluminum and titanium) represent x b$ for this aerospace manufacturer. He wants to reduce their consumption with a target of x00 M$.
Frame contracts with material providers enable to secure availability & prices, but the volume is spread through the full supply chain (only 15% directly purchased)
3 major levers are identified
- secure & control forecast
- optimize nesting
- control recycling value
Results
20% to 30% reduction are confirmed depending on material.
Full digitalization possibility is leveraged by developing nesting algorithms (mono-part nesting and multi-part nesting) with consideration of several constraints
- engineering data (3D models, stress and fatigue constraints)
- manufacturing rules (clamping, batch sizes)
- production plan and production rates
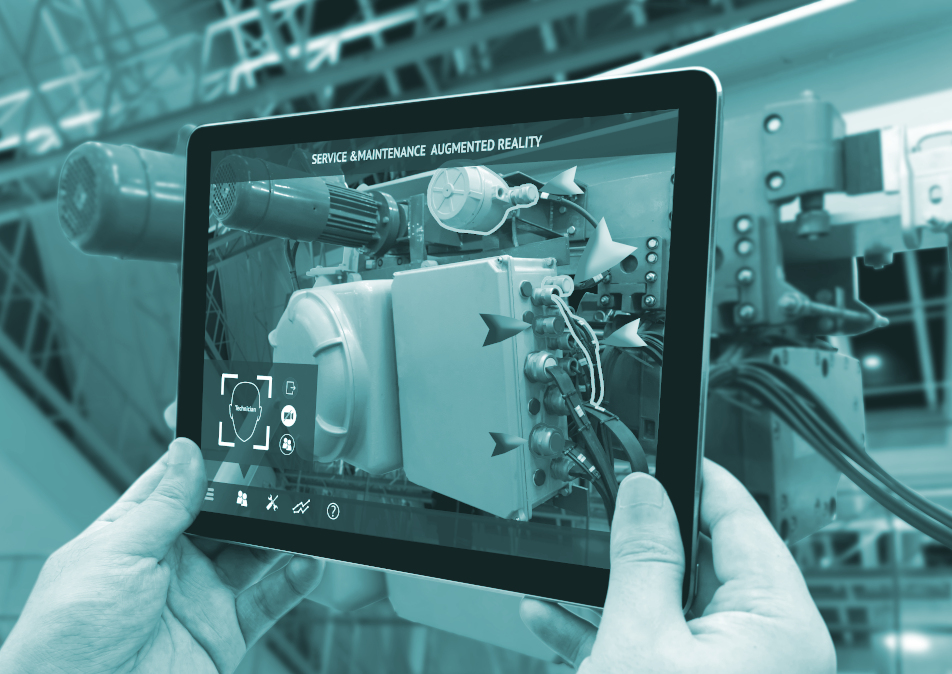